The Value of Machine Learning / Artificial Intelligence, Applied to Shoppers
- Replenium
- Mar 17, 2022
- 3 min read
Updated: Jun 22, 2022
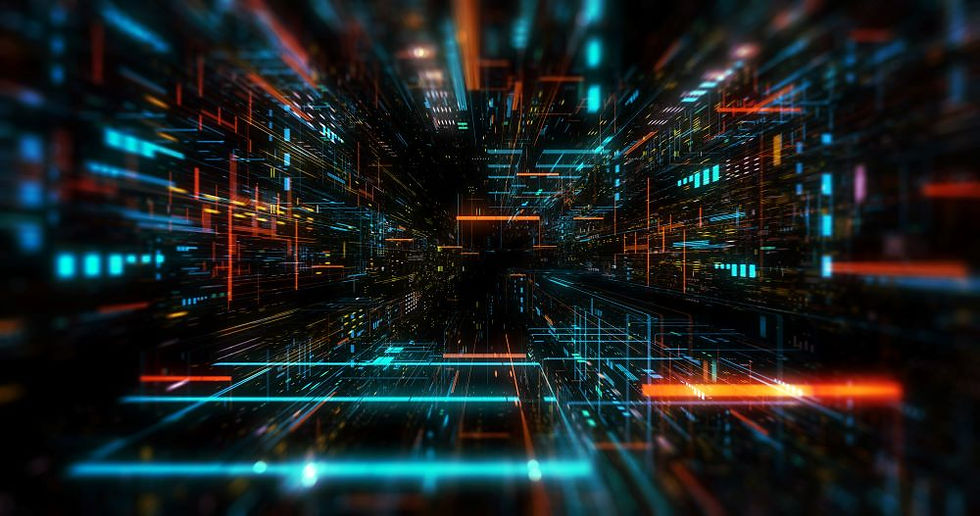
If you were to ask anyone in the general population what the definition of Machine Learning is, you would get a smattering of completely different perspectives as well as quite a few wrong answers. Given the large role technology plays in the market, in businesses, and in our daily lives, machine learning is arguably one of the most leveraged technologies that is understood the least by its user base. That’s a very interesting outlook since in the present, machine learning can be found almost everywhere in the world today. One of the simplest definitions of machine learning is where the machine learns a skill-set by continuously processing copious amounts of data and information. In other words, the machine can learn a skill-set through a high rate of trial and error.
Let's look at an example: one of the first ever instances of machine learning was in 1952 when Arthur Samuel created a computer program that taught itself how to play checkers. The world was in a state of awe as the computer program got better and better at checkers the more and more it played. From the machine’s perspective, it learned by gathering more data and information each time it played. This became the origin and foundation of how people and businesses construct machine learning today.
The evolution of machine learning has been expedient; 1952 was the first case of machine learning, and now (2022) in just 70 years, there is a machine that can play chess and has yet to be beaten by the greatest human chess players in the world.
It’s important to note that machines are not the best at doing everything or more than a few tasks well, they are more or less automated “savants” that learn to excel with one design or action. As we’ve seen over the last decade, multiple companies have developed machines to perform simple tasks extremely well: Siri/Amazon/Cortana (Apple/Amazon/Microsoft) can interpret commands and improve over time at speech recognition. Tesla is making cars that can drive themselves. Machines can also improve with image recognition which has significantly advanced medical technology in terms of identifying disease or cancer at much faster rates. In conclusion, machines are becoming an essential part of society as they’ve helped humanity accelerate its evolution in a variety of ways.
So how does Replenium use machine learning to serve its shoppers? As we’ve seen with other examples of machine learning, the best cases are when the machine masters one technique. With Replenium’s machine learning, the technique is simple, to shop!
Replenium’s machine learning technology helps keep households stocked with essentials and typically at a 10% discount so always for the best prices! As shoppers leverage Replenium’s Auto-Replenishment Platform, they are able to shop for their essential items as well as select a frequency for how often they we will need a given item. Over time, the machine learning capabilities start to pick up on shopping behaviors, both how much a shopper needs as well as how often the shopper needs the item. When you do the math, the amount of time and money saved having a machine helps you keep your essentials stocked adds up greatly over years. Imagine every time you need to go shopping for household essentials, and an assistant will pre-fill your shopping cart with all of your necessities at discounted rates. This allows shoppers the ability to immediately checkout with all their items or allows them to spend more time shopping for what they love or discovering new items.
To learn more about the benefits of using Replenium’s Auto-Replenishment Platform technology, click here.
Brett played baseball at Dartmouth and had aspirations to play professionally but began a financial career instead as an equity trader where he garnered an incredible amount of knowledge with regards to market. He then pivoted into technology after getting an MS in Computer Science.
Comments